Strategies to Navigate Enterprise AI Challenges
Ensuring Accuracy and Dependability in Enterprise AI
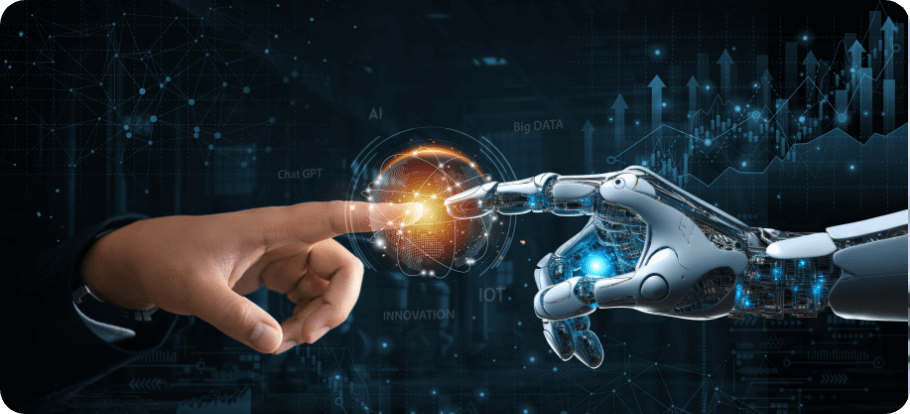
Artificial Intelligence (AI) is increasingly being integrated into enterprise solutions, driving innovation and transforming the way businesses operate. However, as AI is deployed in knowledge worker environments, a couple of unique challenges emerge viz., the puzzling “hallucination effect” and the critical problem of “information reliability”.
Given the increasing scope and use of AI in the enterprise information matrix, both these challenges merit a closer examination.
Understanding the Hallucination Effect in AI
Reliability Challenges in Enterprise AI
Strategies to Address the Hallucination Effect
Improving AI Reliability in Enterprise Settings
What is the Hallucination Effect in AI?
Common Reliability Challenges in Enterprise AI
Addressing the Hallucination Effect in Enterprise AI
Enhancing Reliability in Enterprise AI Systems
What is Hallucination Effect?
The hallucination effect in AI refers to the phenomenon where AI systems generate seemingly plausible yet completely fictitious information. This happens when AI algorithms, in their quest to provide insights or predictions, extrapolate beyond the available data. This can lead to deceptive or erroneous conclusions, at times with serious consequences for the business.
The hallucination effect can be especially troubling in knowledge worker environments, where decisions are made based on the information provided by AI systems. Imagine a data analyst relying on AI-generated insights to inform a critical business strategy, only to discover that these insights were based on data which was erroneous or non-existent. Such events can quickly erode trust in AI systems and prevent their adoption.
Information Reliability Challenge
Closely intertwined with the hallucination effect is the challenge of information reliability. In the age of AI, businesses are inundated with data from a variety of sources. Ensuring the accuracy and trustworthiness of this data is paramount, especially when it forms the foundation for decision-making processes.
Reliability issues can arise at multiple stages of data integration and analysis. Inaccurate data input, biased training data, or errors in AI algorithms can all contribute to unreliable information technology. For example, if an AI model is trained on biased historical data, it can perpetuate and even exacerbate existing biases in its predictions, leading to unfair or discriminatory results.
Additionally, the reliability of AI-generated insights can be compromised if the algorithms lack transparency or interpretability. Decision-makers may be hesitant to act on AI recommendations if they cannot understand how those recommendations were arrived at or if the rationale behind them remains hidden in a “black box”.
Navigating the Challenges: Success Strategies
While the hallucination effect and information reliability challenges may seem daunting, there are several strategies, enterprise can employ to address them and harness the power of AI in organization settings.
- Robust Data Governance: Establishing comprehensive data governance practices is the foundation for addressing both challenges. This includes data quality testing, data lineage tracking, and ensuring that data resources are well-managed. By having strong data governance mechanisms, businesses can mitigate the risk of unreliable data inputs.
- Algorithmic Transparency: Developing AI models with transparency in mind can go a long way in addressing hallucination effect and information reliability challenges. To this end, choosing algorithms that are interpretable and allow for the inspection of decision-making processes is crucial. This will not only enhance trust but also facilitate debugging and error correction.
- Continuous Monitoring: Implementing tracking systems that regularly evaluate the performance of AI models in real-world situations is critical too. Detecting and rectifying instances of the hallucination effect early can prevent major business disruptions.
- Diverse Training Data: Data diversity, including the use of Representative Data Sets, holds the key to ensuring that AI models produce unbiased outputs. By training them on a wide range of data that reflects the real world, we can mitigate bias inherent in any single source. Implementing bias detection and mitigation strategies to counteract bias present in the data can further increase the reliability quotient of the information.
- Human-AI Collaboration: A “collaborative” approach wherein knowledge workers and AI systems work together is recommended instead of completely automated structures. Encouraging people to carefully scrutinize AI-generated insights and incorporating their domain knowledge into the decision-making process will yield more acceptable results.
- Ethics and Compliance: Building clear ethical guidelines and compliance frameworks for AI use within the company may not seem urgent given that enterprise AI is still a maturing concept, having certain guidelines can ensure that AI applications adhere to legal and ethical standards thereby reducing the risk of unreliable or unethical outcomes.
- Education and Training: Investing in education programs to enhance the Data and AI literacy of knowledge workers can pay rich dividends in the long run as equipping them with the skills to understand and assess AI-generated insights can improve the reliability of decision-making.
In conclusion, while the hallucination effect and information reliability challenges pose significant hurdles to the seamless integration of AI in enterprise environments, they are not insurmountable. With a proactive approach to data governance, transparency, tracking, and ethical considerations, businesses can navigate these challenges and unleash the full potential of AI for informed, data-driven decision-making. By addressing these issues head-on, businesses can ensure that AI becomes a prized asset rather than a source of confusion or uncertainty in their quest for innovation and fulfilment.