Generative AI systems have remarkable potential to transform industries through advanced content and automation capabilities. However, these systems do not come cheap and can pose significant computational cost challenges that can deter even the most willing of GenAI advocates. In this article, we’ll look at computational cost challenge of GenAI and examine techniques and solutions that can optimize efficiency and affordability without compromising performance.
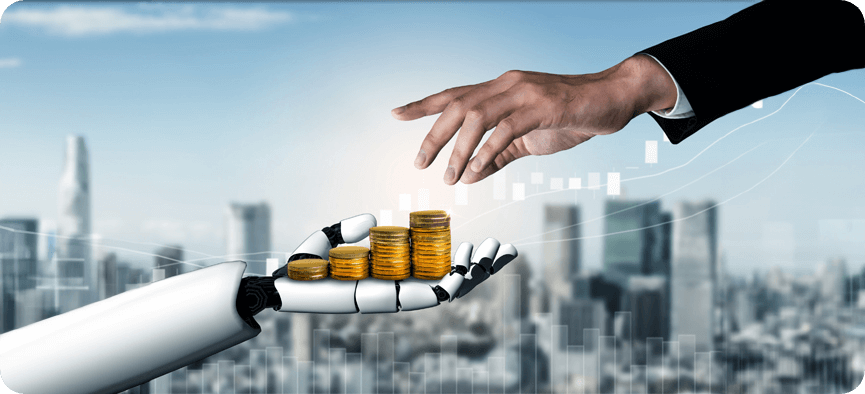
The Computational Cost Conundrum
Generating content and automation with AI demands robust infrastructure like high-speed GPUs and processors. These hardware requirements translate into high costs, which can be a significant barrier to entry for many organizations, especially the ones operating in resource-constrained environments.
That said, one shouldn’t let the cost challenge obstruct AI innovation. With the right strategies, Generative AI systems can be made cost-effective without compromising capabilities.
Balancing Efficiency with Affordability
When it comes to strategies for addressing the computational cost challenges, it is best to find a balance between efficiency and affordability. We recommend the following techniques to manage cost equation better.
- Model optimization can enhance computational efficiency without sacrificing performance. This can be achieved via Quantization and Parameter-Efficient Fine-Tuning (PEFT), two techniques which help reduce the precision and size of the model respectively. While Quantization reduces the number of bits used to represent each number in the model, PEFT focuses on adapting large pre-trained models to specific downstream tasks by fine-tuning only a small subset of their parameters. This significantly reduces the computational cost and memory footprint compared to traditional fine-tuning.
- Cloud-based Solutions: Leveraging cloud-based AI platforms can provide organizations with a scalable and cost-effective way to manage computational costs. Cloud platforms offer access to a wide range of computing resources on-demand, which can be scaled up or down as needed. This eliminates the need for organizations to invest in and maintain their own infrastructure. Besides, the added advantage of pay-as-you-go pricing models mean that organizations only pay for the resources they use.
- Model Caching: Specifically targeted at inference time, model caching offers a strategy to address the computational cost challenges associated with GenAI. This approach involves storing previously generated content whenever possible, eliminating the need for redundant computation. By leveraging a cache, organizations can preserve frequently generated outputs, saving time and valuable computational resources during the inference stage. This effectively reduces the workload on the AI system, leading to both conserved resources and enhanced responsiveness.
Harnessing the Power of Open-Source Models
One of the main challenges organizations face in deploying generative AI systems is the potentially high computational costs. Licensing fees, ongoing subscriptions, and the demand for high-end hardware can quickly add up and running proprietary models can indeed get very expensive, very fast. One way to make generative AI more affordable is to use open-source models and fine-tune them for specific needs. Open-source models are freely available and can be deployed on a variety of hardware platforms, making them much more cost-effective than proprietary models.
Fine-Tuning Open-Source Models and LoRA & QLoRA Advantage
Fine-tuning is a process of adjusting the parameters of an existing model to improve its performance on a specific task. By fine-tuning an open-source model, organizations can create a custom model that meets their specific needs without incurring the high cost of a proprietary model.
At USEReady, we offer our customers two innovative frameworks viz., LoRA and QLoRA which enable organizations to fine-tune open-source models, such as LLaMA 2, to maximize their efficiency and affordability.
LoRA or Low-Rank Adaptation is a technique that focuses on adapting a pre-trained, open-source model by using a low-rank approximation. By applying this approach, organizations can significantly reduce the computational demands of the model, making it possible to run these large models on more affordable hardware. The result is a cost-effective solution that doesn’t compromise on performance.
QLoRA, short for Quantization Low-Rank Adaptation, combines the advantages of both quantization and low-rank adaptation. This innovative approach involves reducing the numerical precision of the model (quantization) while simultaneously applying low-rank adaptation. The synergy between these techniques not only reduces computational costs but also conserves memory resources, making it a powerful tool for organizations seeking cost-effective AI solutions.
Integrating Fine-Tuning into Your Strategy
When considering the deployment of generative AI systems, it’s essential to factor in the cost-efficiency of the models you choose. Fine-tuning open-source models using LoRA and QLoRA can be a game-changer for your organization, particularly if you’re operating with limited budgets.
The process involves adapting the model to your specific requirements and objectives, ensuring that it understands the nuances and jargon of your industry. Fine-tuned models not only perform better but also consume fewer computational resources, which is key to managing costs.
To make the most of this cost-saving strategy, consider the following steps:
Managing the computational costs of powerful generative AI systems is key for wider adoption. The strategies discussed can optimize efficiency and affordability without sacrificing capabilities. With the right techniques, organizations can benefit from AI’s immense potential while reducing expenses. Fine-tuning provides access to advanced generative AI in a fiscally responsible way, enabling continued innovation and proliferation of GenAI across the enterprise.